Interpreting Results of a Hypothesis
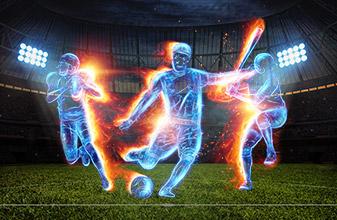
Sharp Sports Betting is a tool for those interested in winning money at sports betting. The book explains the most common sports bets, what all the numbers mean, and the mathematics behind the numbers.
Let's go over basic sports betting fundamentals. All sports bettors should learn the following facts. Every other type of casino advantage player, including blackjack players of all skill levels, should have a functioning understanding of Expected Value. You should not be betting online or at land-based casinos, such as in Las Vegas, without this understanding.
You formulated your hypothesis
You use it to make predictions about games that were not used in the formation of the hypothesis. Now what? A full explanation of the science of statistics is beyond the scope of this article. If this area intrigues you, I suggest taking a college course in statistics.
This article discusses only one tiny area of statistics: using the binomial distribution to examine the significance of W-L records.
You have a win-loss record that describes your sample. You are trying to show that the W-L record of your sample is significantly different from 50 percent wins.
Two Standard Errors
The most generally used standard of statistical significance is five percent. As a close approximation, five percent rarity occurs when the W-L record of the sample you have gathered is two standard errors different from 50 percent wins.
The square root of your sample size is the standard deviation of the difference between your total wins and total losses, which I will call excess wins.
The easy way to find the number of standard errors is to divide excess wins by the standard deviation. You have reached that five-percent point when the your excess wins is two standard errors.
For example, suppose you tested the hypothesis that NFL home dogs of +7 or more are good bets by examining the games played during the 2020-2021 seasons. Suppose you came up with a W-L record of 30-25.
That’s a sample size of 55 decisions. The square root of 55 is 7.4. 30 wins minus 25 losses is 5 excess wins. That’s less than one standard error. For 55 decisions you need a W-L record of 35-20 to have statistical significance at the five percent level.
Suppose the W-L record for NFL home dogs of +7 or more was 32-15 for games played during 2016-2019. If you add those games in, you get a record of 62-37 for the six-year period 2016-2021. Can you call that a sample of 99 decisions and test for significance?
No you cannot do that. The reason is you used those 2016-2019 games to formulate and modify your hypothesis. You cannot also use them to test the hypothesis. Only the games played in years other than 2016-2019 can be used to test that hypothesis.
Two Standard Errors is Too Few
Two standard errors is enough significance for many purposes, but not for betting sports.
The search for profitable betting systems involves examining large numbers of possible relationships. Approximately five percent of the relationships you examine will meet a two-standard-error test by chance alone. Examine a hundred possible relationships all of which are really no relationship, and you likely will find five that are significant at the two-standard-error level.
The two-standard-error standard is reasonable to use for testing non-betting applications where there is only one hypothesis being tested, and that hypothesis is never modified. But if dozens of hypotheses are being tested, or if you are willing to modify your hypothesis, then two standard errors gives too many false positives.
There are publications that report results that look like relationships but are in fact the expected product when one examines large quantities of random data. The process of looking for relationships where most likely none exist is called data mining. The results of data mining are called angles. Data mining can turn up nuggets, and some angles can work. However, most of what look like strong relationships in past data describe only the past data, and fail in attempts to predict the results of future games.
If you have discovered a relationship that really exists, then examining more and more games will result in the level of significance going up and up. If the first 100 games you analyze give you a 60-40 record, you are at two standard errors. So keep gathering more data. By the time your sample size gets to 200, your W-L record might be 120-80 whereas the requirement for two standard errors is a W-L record 114-86.
As your sample size increases, if the added games seem to have just 50 percent winners, then your initial results that made you so hopeful were likely a random blip in the data and do not result in a system you can use to win money from sportsbooks.
If you are testing hypotheses using games already played rather than games yet to be played, you must use a higher standard of significance than just five percent rarity. If you are going to draw conclusions from data mining but not test your hypotheses against different data, then the minimum requirement for statistical significance is four standard errors. Think of it as two standard errors to develop the hypothesis and then two more to test it.
If you are testing a system against games already played, my suggestion is to hold out for 1:1000 chance of a win-loss record being achieved by chance alone. Even with a standard that high you will occasionally find false positives, methods that you at first think find good bets but later prove to be worthless.
This is part of an occasional series of articles.
Excerpted with permission from Sharp Sports Betting by Stanford Wong, edited for this format
Please log in or register to leave a comment